We strive to address one of the world’s most complex problems: drug discovery.
New drug development cost: $2.6 billion. Success rate: below 10%.
One of the first phases of drug discovery for a chosen target concerns high-throughput screening. Machine learning has the potential to substantially accelerate the drug discovery lifecycle by performing virtual screening of large databases of candidate drug molecules against a specific target a protein associated with some known disease. At the same time current approaches for structure-based virtual screening are slow.
VALUE PROPOSITION
Our unique proposition is a Virtual Screening as a Service (VSaaS) solution.
We propose a service for fast virtual screening, suitable for massive databases with minimal requirements.
DeNViS can be employed for tasks within early-stage drug discovery: drug repurposing, repositioning and potentially other applications within the drug discovery pipeline.
Why us?
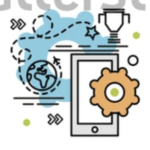
104x speed-up compared to state-of-the-art docking-based approaches such as gina, vina etc. DeNViS can be employed to screen billions of compounds in a matter of hours.
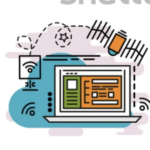
State-of-the-art performance employing algorithms in geometric deep learning, outperforming docking-based approaches in binding affinity prediction tasks evaluated in multiple benchmarks.
What our collaborators say about DeNViS
"Leveraging its extensive deep learning expertise, DeepLab's virtual screening approach is a novel, high throughput methodology in binding affinity elucidation. Fueled by state-of-the-art machine learning methods, this approach is propelling drug-repurposing into an exciting future."
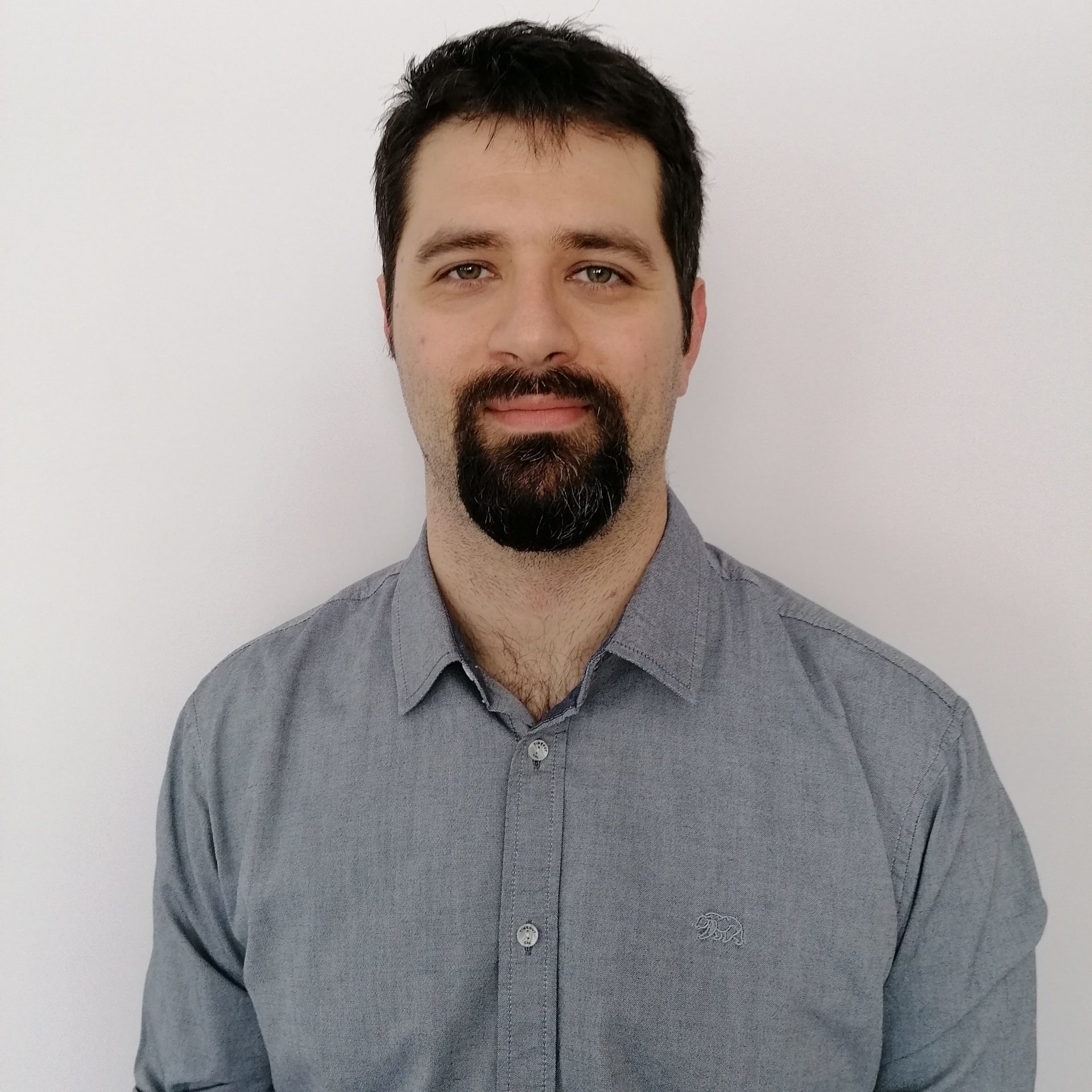
Stefanos Leptidis
Postdoctoral Researcher in Cardiac Transcriptomics & Data Analysis
"DeepLab enables you to reimagine how healthcare is delivered. By using state of the art scientific approaches to drug discovery, we apply ideas that are novel and life-changing!"
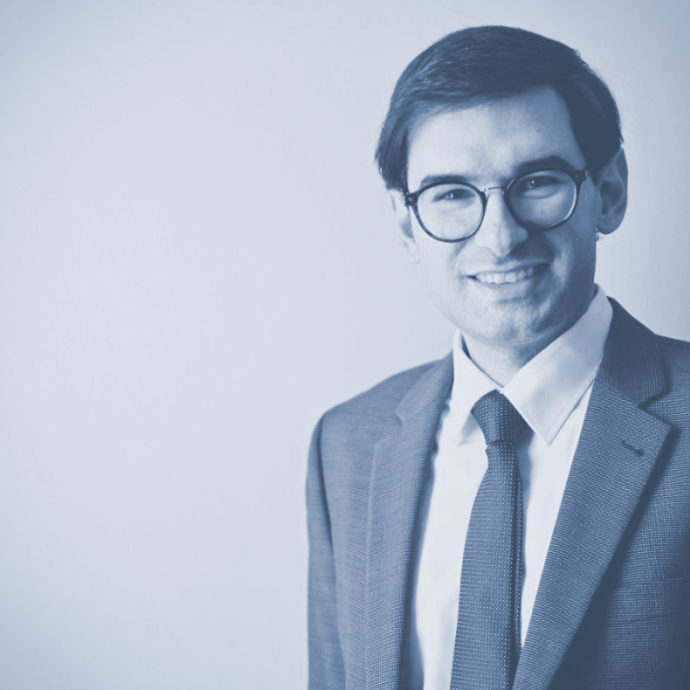
Efthymios Vogiatzis
HealthTech Business & Market Analysis Consultant
Publication:
- A community effort to discover small molecule SARS-CoV-2 inhibitors, (under submission) 2023, [preprint]
- Α. Krasoulis, N. Antonopoulos, V. Pitsikalis, S. Theodorakis, "DENVIS: Scalable and high- throughput virtual screening using graph neural networks with atomic and surface protein pocket features", Journal of Chemical Information and Modeling (in press) 2022, [doi, preprint]